George Coyle and I have collected and published what we believe are some of the most interesting takeaways from “The Man Who Solved the Market” – a great book about Jim Simons and Renaissance Technologies (RenTec) authored by Gregory Zuckerman. You can read about those takeaways HERE in part 1, and HERE in part 2.
In light of the popularity of part 1 and part 2, we decided to share our thoughts on what we believe RenTec may actually be doing. A lot of this was covered in the previous quotes, but this article includes thoughts, opinions, narrative, and added color based on experience.
We hope you’ll enjoy reading this part 3. Subscribe HERE if you’d like to receive our future publications.
Part 3
Decoding RenTec
Written by George Coyle, edited by Moritz Seibert
As I have studied RenTec with a reasonable degree of attention to detail (after having published part 1, Gregory Zuckerman said that we read his book more closely than his editor!), and since I’ve spent many years building all kinds of quant models, I’ve developed what I feel is a reasonably educated guess of what lives behind the RenTec curtain. The purpose of this article is to share those thoughts. Few caveats:
- Everything that follows is my opinion. I have no “inside” information.
- My assumption is the information gathered by various reporters, especially by Zuckerman, is accurate. This is a critical assumption. I believe it is a reasonable assumption though since the RenTec “story” remains much the same over time when you connect the dots from what few articles and interviews exist.
- If it turns out that I am right, you’d need a lot of AUM to diversify appropriately. You’d also need a lot of money to build out the infrastructure and you’d need terrific banking connections to get swaps enabling you to set up highly leveraged positions with low costs that also allow preferential tax treatment (and probably fancy lawyers to plead your case to the IRS in court).
- Any attempt to reduce everything to this many pages is not a full account. If you want the whole story, read the book!
Beyond that, and before we go further, I must share the following: If by chance someone at RenTec reads this and thinks, “Hey, this George guy gets it, we should hire him or give him a piece of the Medallion fund to shut him up” I’d gladly retract this article and erase my “memory” in exchange for a seat and/or a piece of the Medallion fund. Feel free to call, day or night!
That said, let’s get into it using quotes from The Man Who Solved the Market (TMWSTM).
First, I’d like to establish what RenTec is not. Despite many rumors I’ve heard over the years, according to TMWSTM, RenTec is not a high frequency trader (HFT).
Staffers became excited about developing super- short- term signals to trade in a matter of seconds, or even less, a method that would become known as high- frequency trading. Renaissance’s computers proved too slow to beat others to the market, however.
We also know that they had a lot of positions on, and that they hedged or built positions in ways which made them look like a HFT firm (likely resulting in the HFT confusion):
Medallion still held thousands of long and short positions at any time, and its holding period ranged from one or two days to one or two weeks. The fund did even faster trades, described by some as high- frequency, but many of those were for hedging purposes or to gradually build its positions… Medallion made between 150,000 and 300,000 trades a day, but much of that activity entailed buying or selling in small chunks to avoid impacting the market prices, rather than profiting by stepping in front of other investors…
That established, it is important to know they kept really good data from the get-go:
No one had asked Straus to track down so much information. Opening and closing prices seemed sufficient to Simons and Ax…No one had told Straus to worry so much about the prices, but he had transformed into a data purist, foraging and cleaning data the rest of the world cared little about.
This is important because, with modeling like many things, it is garbage in garbage out. In other words, if you feed bad data to a model, the results will also be bad since the data is not accurate. We will revisit this shortly.
From there, we know RenTec started out as long-term futures traders with fairly simple models (note: Axcom = RenTec’s name early on):
For all the brainpower the team was employing, and the help they were receiving from Carmona and others, Axcom’s model usually focused on two simple and commonplace trading strategies. Sometimes, it chased prices, or bought various commodities that were moving higher or lower on the assumption that the trend would continue. Other times, the model wagered that a price move was petering out and would reverse, a reversion strategy…Axcom often held on to investments for weeks or even months at a time.
And they migrated to shorter-term futures trading:
…Berlekamp argued, because markets can be volatile. Infrequent trading precluded the firm from jumping on new opportunities as they arose and led to losses during extended downturns. Berlekamp urged Ax to look for smaller, short- term opportunities— get in and get out…Ax had resisted shifting to a more frequent, short- term trading strategy, partly because he worried brokerage commissions and other costs resulting from a fast- paced, higher- frequency approach would offset possible profits…Ax showed Berlekamp a letter he’d received from Simons’s accountant, Mark Silber, ordering Axcom to halt all trading that was based on the firm’s struggling, longer- term predictive signals until Ax and his team produced a plan to revamp and improve its trading operations…One day, Berlekamp presented Ax with an idea. “Why don’t I buy your stake in the firm?”
As to what they were doing:
Their goal remained the same: scrutinize historic price information to discover sequences that might repeat, under the assumption that investors will exhibit similar behavior in the future…Make a lot of trades, however, and each individual move is less important, reducing a portfolio’s overall risk…Sifting through Straus’s data, Laufer discovered certain recurring trading sequences based on the day of the week…Simons and his researchers didn’t believe in spending much time proposing and testing their own intuitive trade ideas. They let the data point them to the anomalies signaling opportunity. They also didn’t think it made sense to worry about why these phenomena existed. All that mattered was that they happened frequently enough to include in their updated trading system, and that they could be tested to ensure they weren’t statistical flukes…They did have theories. Berlekamp and others developed a thesis that locals, or floor traders who buy or sell commodities and bonds to keep the market functioning, liked to go home at the end of a trading week holding few or no futures contracts, just in case bad news arose over the weekend that might saddle them with losses. Similarly, brokers on the floors of commodity exchanges seemed to trim futures positions ahead of the economic reports to avoid the possibility that unexpected news might cripple their holdings. These traders got right back into their positions after the weekend, or subsequent…“We’re in the insurance business,” Berlekamp told Straus. Oddities in currency markets represented additional attractive trades. Opportunity seemed especially rich in the trading of deutsche marks. When the currency rose one day, it had a surprising likelihood of climbing the next day, as well. And when it fell, it often dropped the next day, too. It didn’t seem to matter if the team looked at the month- to- month, week- to- week, day- to- day, or even hour- to- hour correlations; deutsche marks showed an unusual propensity to trend from one period to the next, trends that lasted longer than one might have expected….They [certain trade signals] were so faint, the team took to calling them ghosts, yet they kept reappearing with enough frequency to be worthy additions to their mix of trade ideas…Simons had come around to the view that the whys didn’t matter, just that the trades worked…“Volume divided by price change three days earlier, yes, we’d include that,” says a Renaissance executive. “But not something nonsensical, like the outperformance of stock tickers starting with the letter A.”
While skipping ahead a bit (relative to the book) in the name of keeping this article’s narrative tight, over time, they altered the process:
To make it all easier to digest, they had broken the trading week into ten segments— five overnight sessions, when stocks traded in overseas markets, and five day sessions. In effect, they sliced the day in half, enabling the team to search for repeating patterns and sequences in the various segments. Then, they entered trades in the morning, at noon, and at the end of the day…eventually deciding five-minute bars were the ideal way to carve things…Laufer’s five-minute bars gave the team the ability to identify new trends, oddities, and other phenomena, or, in their parlance, nonrandom trading effects…One early discovery: Certain trading bands from Friday morning’s action had the uncanny ability to predict bands later that same afternoon…It wasn’t immediately obvious why some of the new trading signals worked, but as long as they had p-values, or probability values, under 0.01—meaning they appeared statistically significant, with a low probability of being statistical mirages—they were added to the system.
This is a good point to digress. As those who have spent time backtesting know, it is pretty easy to create epic short-term systematic backtest returns that would make you rich beyond your wildest imagination. One of the main problems lies in getting the executions/trade fills. I will explain.
Let’s say you have a system with a limit price buy order (aka you want to buy at $100 but the market is >$100 so you will buy if/when the market gets to $100). When backtesting, if you are inexperienced, you may just assume you got an execution if the market traded at your price. When you put the model in the actual market, you quickly learn this doesn’t always prove true. Sometimes, your limit orders are at the back of the order book and don’t get filled. Let’s say you place a limit order to buy XYZ at $100. Let’s further say you are the 95th person in the order book. Specific details of how exchanges work aside, if 94 shares trade, you won’t get filled – you were too far back in the line.
The problem doesn’t stop with limit orders. If you use a stop order (buy/sell at the market when the price is reached), you usually encounter slippage. But it sounds like RenTec may not have these problems per TMWSTM:
- Profitable trade ideas are only half the game; the act of buying and selling investments can itself affect prices to such a degree that gains can be whittled away.
- From the earliest days of the fund, Simons’s team had been wary of these transaction costs, which they called slippage. They regularly compared their trades against a model that tracked how much the firm would have profited or lost were it not for those bothersome trading costs…They called it The Devil [difference between model outcome and real outcome].
- …began developing sophisticated approaches to direct trades to various futures exchanges to reduce the market impact of each trade. Now Medallion could better determine which investments to pursue, a huge advantage as it began trading new markets and investments.
- Having first sent orders to a team of traders five times a day, it eventually increased to sixteen times a day, reducing the impact on prices by focusing on the periods when there was the most volume.
So, RenTec spent a lot of time, energy, and probably money making sure they got the fills. And this dovetails with the comments above about HFT – they looked like HFT guys but really, they were just piecing out orders to make sure “The Devil” was near zero (or so it seems). As per above and at the risk of being redundant, if you can get the executions on short-term models as expected (aka The Devil = zero), you can print money. They trade short-term models and mostly print money so, yeah.
Rhyming with the comments above, data played a large part according to TMWSTM:
…they wielded a big advantage: They had more accurate pricing information than their rivals.
Things were going swimmingly but Simons was looking for the next thing to enable RenTec to be able to make 80% a year. As TMWSTM put it:
Jim Simons seemed to have discovered the perfect way to trade commodities, currencies, and bonds: predictive mathematical models. Yet, Simons knew, if he wanted Renaissance Technologies to amount to much of anything, he’d have to get his computers to make money in stocks.
Meanwhile, something was afoot at Morgan Stanley which would provide the fuel to allow RenTec to achieve Simons’s goal per TMWSTM:
A tall, trim computer-science graduate from Columbia University, Bamberger provided analytical and technical support for Morgan Stanley’s stock traders, serving as an underappreciated cog in the investment bank’s machine…When the traders prepared to buy and sell big chunks of shares for clients, acquiring a few million dollars of Coca-Cola, for example, they protected themselves by selling an equal amount of something similar, like Pepsi, in what is commonly referred to as a pairs trade…Each time, the trading activity altered the gap, or spread, between the stock in question and the other company in the pair, even when there was no news in the market…Once the effect of their Coke stock selling wore off, the spread between the shares reverted to the norm, which made sense, since there had been no reason for Coke’s drop other than Morgan Stanley’s activity…Bamberger sensed opportunity. If the bank created a database tracking the historic prices of various paired stocks, it could profit simply by betting on the return of these price-spreads to their historic levels after block trades or other unusual activity…It wouldn’t be clear for many years, but Morgan Stanley had squandered some of the most lucrative trading strategies in the history of finance.
This is a good point for another digression. Having been an execution trader for big fundamental long/short or market neutral hedge funds for several years, I know that hedging risk on “block” trades with highly correlated stocks (or baskets of stocks) happens at banks. The banks often facilitate the block trades with “capital” (aka their own money) and, not wanting to lose, they often hedge their position in a related stock or sector until they get out of their capital position (or it certainly used to happen).
Anyway, eventually Morgan Stanley’s Robert Frey joined RenTec and ran a long/short portfolio which didn’t do so well:
Frey’s models usually just focused on whether relationships between clusters of stocks returned to their historic norms…The problem wasn’t that Frey’s system couldn’t discover profitable strategies. It was unusually good at identifying profitable trades and forecasting the movement of groups of shares. It was that, too often, the team’s profits paled in comparison to those predicted by their model. [The Devil]
As to what was wrong:
It turned out that Frey’s model made suggestions that were impractical, or even impossible…when Nova’s [Frey’s fund/model] leverage crossed a certain threshold, Frey and staffers manually shrank the portfolio to remain within the necessary limits, overriding their model’s recommendations…bet against, certain stocks that weren’t actually available to be sold, so Frey had to ignore the recommendations…futures trading was simple stuff; if a trade didn’t happen, there were few consequences. With Frey’s stock-trading system, failing to get just a few moves done threatened to make the entire portfolio more sensitive to market shifts, jeopardizing its overall health.
RenTec had previously hired now TMWSTM famous Brown, Mercer, and Magerman who joined the stock group. They found some bugs in the stock trading code. They fixed the bugs while solving for The Devil and the models made money.
Here again it sounds like good data and solving for the inability of a model to meet reality (aka The Devil) was a big part of the success. Further, it does not sound like the models themselves were particularly amazing or groundbreaking (despite reports in the press).
From there, they rolled everything up into one big fund according to TMWSTM:
…Simons to merge the Nova fund [stocks] into Medallion [futures] , creating a single hedge fund trading almost every investment.
Let’s pause and summarize. Per above, the Medallion fund is likely something of a portfolio of short-term futures strategies and short-term statistical arbitrage strategies. But it does not stop there.
The next thing to consider is RenTec’s rough patch in the year 2000 as several interesting things emerge from this period:
Medallion faced inexplicable losses. It lost about $90 million in a single day in March; the next day it was $80 million more. Nerves began to fray—until then, Medallion had never lost more than $5 million in a day…because so many of the system’s trading signals had developed on their own through a form of machine learning, it was hard to pinpoint the exact cause of the problems…After several more all-nighters, a couple of researchers developed a theory about what was causing the problems: A once-trusted strategy was bleeding money. It was a rather simple strategy—if certain stocks rallied in previous weeks, Medallion’s system had taught itself to buy more of those shares, under the assumption the surge would continue. For several years, this trending signal had worked, as the fund automatically bought Nasdaq shares that were racing still higher. Now the system’s algorithms were instructing Medallion to buy more shares, even though a vicious bear market had begun…They quickly ditched the momentum strategy, stemming the losses. Soon, gains were piling up once again.
First, it is very telling it took them days to figure out where the losses were coming from. Think about that, if you have a portfolio and you are losing money, it usually takes about 10 seconds to see where the losses are coming from (you log into the brokerage account, sort by largest loss, done). In RenTec’s case per above, it took days to figure it out.
As a system builder, and as TMWSTM states, the conclusion is that they have a model running the models and there are likely a lot of overlapping models trained on the same markets. My guess is they probably have some centralized risk system that dictates risk limits. Stated another way, let’s say they have 50 models, and all models indicate they should buy AAPL at the same time. My guess is they don’t let that happen (or their ML/AI which is likely bounded by humans due to risk considerations doesn’t let it happen). They say AAPL can only get so big or only 10 of 50 models can be long at once. Which 10 models would depend on many contingencies (which models were on, which were the highest probability, etc.). This would explain why it would take days to figure out which models were causing problems – you’d have to sift through which models were doing what and which overrides were on/off amidst the massive body of computer code. I can’t think of any other reason it would take days to figure this out. If you have an idea, please share.
Although, and in the name of a little comic relief with a dense topic, it would be great if RenTec’s collective “kryptonite” (Superman reference) was an inability to see losses brought on by so many years of never seeing one. This fun thought reminds me of the following Larson cartoon.
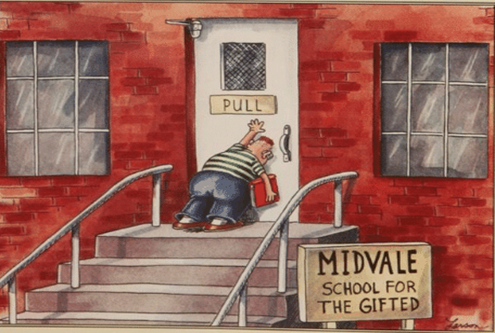
Jokes aside, the hints from this period go beyond just the above inference, and this is where model building experience is also helpful. Some quant strategies (e.g., trend following) contend with a future that does not look like the past and manage the potential for things going off the rails using stop losses. Trend models might get whipsawed, but they wouldn’t keep buying the dip – new highs would be needed to get in again. As per the book, the tech stocks kept falling (“…vicious bear market…”) and RenTec kept losing (aka long in a downtrend). As to how momentum models could be long in a bear market/downtrend, my short answer guess is bear market rally head fakes.
Other quant methods tend to find historical patterns and qualify models using tests of statistical significance while assuming the same patterns will continue. The challenge with these models is figuring out when they are broken. Pattern analysis types generally solve for these problems with diversification (mentioned in a quote above) and discretionarily adding/deleting component models.
Given the stocks/models referenced above caused so much pain to the portfolio in the year 2000, we can only infer they were in the pattern camp – otherwise they would have been “stopped out.” My assumption/inference here is largely confirmed in TMWSTM in a general sense:
- By 1997, Medallion’s staffers had settled on a three- step process to discover statistically significant moneymaking strategies, or what they called their trading signals. Identify anomalous patterns in historic pricing data; make sure the anomalies were statistically significant, consistent over time, and nonrandom; and see if the identified pricing behavior could be explained in a reasonable way.
- It wasn’t immediately obvious why some of the new trading signals worked, but as long as they had p-values, or probability values, under 0.01—meaning they appeared statistically significant, with a low probability of being statistical mirages—they were added to the system.
Further, a weakness with using pattern models is how to combine and weight them whereas trend systems tend to account for the portfolio level considerations. As stated in TMWSTM:
Simons was challenging them to solve yet another vexing problem: Given the range of possible trades they had developed and the limited amount of money that Medallion managed, how much should they bet on each trade?…Laufer began developing a computer program to identify optimal trades throughout the day, something Simons began calling his betting algorithm.
Another digression, I wonder if they/anyone has used a trend system “governor” on a multitude of pattern models using the equity curves of the individual pattern models like a trend guy uses market prices, buying breakouts, setting stops using ATR multiples on the equity curve of the specific model, etc. Instead of trading a trend system on specific markets, trade it on specific pattern models. If this makes sense to you, please reach out (contact info below) and let’s nerd out on “Frankenstein” quant model ideas.
A final consideration, which I won’t substantiate here but was talked about in the book, is that they explored factor investing.
Let’s pause and recap again. We know the portfolio is short-term. We know it uses hundreds to thousands of futures and statistical arbitrage strategies on lots of different time horizons. We know they consider factor models. We know there is a master model (ML/AI) picking the sub models since TMWSTM said so and this is the only logical explanation on why it would take a gaggle of rocket scientists days to figure out why they were losing money.
Combining all of this and filling in the blanks with experience and hunches, the RenTec component futures/statistical arbitrage models are likely categorized by factors and are governed by some kind of selection “bot” (ML/AI/efficient frontier masquerading as AI) that chooses the portfolio (from thousands of sub models) at fixed intervals using probabilistic (literally) sizing algorithms which are bounded to contend with the AI/ML trying to go “all in” (poker reference) on any one model within a factor bucket. My guess is RenTec has additional governing risk models to prevent the system from too much directional bias. Models on models on models.
Assuming this is roughly right, I imagine the component models are not that complex nor is any part of the system. But when you put it all together, well, lots of simplicity combined becomes incredibly complex.
While there are likely many other facets that would be worth exploring in a room full of quant modelers endowed with the capital to give this a shot, I will stop here with the quant part of things. That said, there are a few other points worth mentioning to the would-be imitator…
Another very interesting aspect of RenTec is they collaborate internally. As the book explains, anyone could add to the models (assuming they actually “could” [aka had the skills] add to the models). According to TMWSTM:
Rival trading firms often dealt with the issue by allowing researchers and others to work in silos, sometimes even competing with each other. Simons insisted on a different approach—Medallion would have a single, monolithic trading system. All staffers enjoyed full access to each line of the source code underpinning their moneymaking algorithms, all of it readable in cleartext on the firm’s internal network…Simons hoped his researchers would swap ideas, rather than embrace private projects.
Why is this important? Because it is different/unusual for the business (as are the RenTec returns).
But their success goes beyond all of the above. Some of their “edge” came from banking relationships, leverage, and advantageous tax structures. How much? Hard to say. But the following quotes provide insight:
- (Just as homeowners take out mortgages to buy homes that are more expensive than they can afford with the money they have in the bank, so too do hedge funds like Medallion, as a way to boost profits, borrow money to accumulate larger investment portfolios than their capital would allow.)…basket options were a crafty way to supercharge Medallion’s returns…When Medallion spied especially juicy opportunities, such as during a 2002 market downturn, the fund could boost its leverage, holding close to $20 of assets for each dollar of cash, effectively placing the portfolio on steroids…securities in the basket-options transactions, the most Medallion could lose in the event of a sudden collapse was the premium it had paid for the options and the collateral held by the banks…One staffer was so shocked by the terms of the financing that he shifted most of his life savings into Medallion, realizing the most he could lose was about 20 percent of his money.
- …basket options: They enabled Medallion’s trades to become eligible for the more favorable long-term capital gains tax…
As I stated at the beginning, and assuming I’m in the ballpark with this exploration, you’d need a whole lot of money and relationships to replicate what they’re doing. I imagine the volume of people/firms in the world with the risk appetite and resources to make this happen is pretty low. Finding a backer for the fully functioning apparatus seems improbable, especially considering there is no guarantee my exploration here is correct. If a reader wants to give us a shot, reach out! That said, all this leads me to wonder if perhaps the RenTec’s of the world can only come into being via gradual evolution. I will leave you to draw your own conclusions.
Thanks again to Gregory Zuckerman for his great book!
If you’d like to reach out and discuss this, please feel free to contact us on Twitter: @moritzseibert and @gfc4
Stay safe and #happytrading.